diff options
author | Nicholas Leonard <nick@nikopia.org> | 2015-09-21 22:12:06 +0300 |
---|---|---|
committer | Nicholas Leonard <nick@nikopia.org> | 2015-09-21 22:12:06 +0300 |
commit | db48bc03c2176df9333783859c7df4bc1a028910 (patch) | |
tree | dcbdd6af5f0432806defd19240fe244a362f90ef | |
parent | 127e7dbe7826e00a7afa38a14c4a8174c5fe97ba (diff) | |
parent | 06a0f3735801c3a8814211237030136bb6b3b4bc (diff) |
Merge branch 'master' of github.com:torch/torch.github.io into rva
-rw-r--r-- | _config.yml | 1 | ||||
-rw-r--r-- | _data/authors.yml | 3 | ||||
-rw-r--r-- | _data/nav_docs.yml | 2 | ||||
-rw-r--r-- | _includes/header_blog.html | 4 | ||||
-rw-r--r-- | _includes/nav_blog.html | 12 | ||||
-rw-r--r-- | _layouts/post.html | 4 | ||||
-rw-r--r-- | blog/_posts/2015-07-11-first-post.md | 8 | ||||
-rw-r--r-- | blog/_posts/2015-07-30-cifar.md | 2 | ||||
-rw-r--r-- | blog/_posts/2015-09-07-spatial_transformers.md | 120 | ||||
-rw-r--r-- | blog/all.md | 7 | ||||
-rw-r--r-- | blog/index.md | 6 | ||||
-rw-r--r-- | docs/02-developer-docs.md | 260 | ||||
-rw-r--r-- | docs/whoweare.md | 2 | ||||
-rw-r--r-- | static/flow.css | 18 |
14 files changed, 388 insertions, 61 deletions
diff --git a/_config.yml b/_config.yml index 1bf19ab..e805ef4 100644 --- a/_config.yml +++ b/_config.yml @@ -2,6 +2,7 @@ baseurl: / url: http://soumith.ch name: Torch +title: Torch relative_permalinks: false markdown: redcarpet redcarpet: diff --git a/_data/authors.yml b/_data/authors.yml index ae4cd50..4d52a72 100644 --- a/_data/authors.yml +++ b/_data/authors.yml @@ -5,3 +5,6 @@ soumith: szagoruyko: name: Sergey Zagoruyko web: http://imagine.enpc.fr/~zagoruys +alband: + name: Alban Desmaison + web: https://moodstocks.com/
\ No newline at end of file diff --git a/_data/nav_docs.yml b/_data/nav_docs.yml index 4057f0d..401ef12 100644 --- a/_data/nav_docs.yml +++ b/_data/nav_docs.yml @@ -10,3 +10,5 @@ title: Tutorials, Demos, Examples - id: package-docs title: Package Documentation + - id: developer-docs + title: Developer Documentation diff --git a/_includes/header_blog.html b/_includes/header_blog.html index 33baeae..a254456 100644 --- a/_includes/header_blog.html +++ b/_includes/header_blog.html @@ -1,7 +1,7 @@ <div class="blog-heading"> <h1> The Torch Blog - </h1> <a href="/blog/feed.xml"> - <img src="http://torch.ch/static/rssicon.svg" width="20"> + <img src="http://torch.ch/static/rssicon.svg" width="20" style="display:inherit; margin: 0;"> </a> + </h1> </div> diff --git a/_includes/nav_blog.html b/_includes/nav_blog.html index 356f94d..e605c0a 100644 --- a/_includes/nav_blog.html +++ b/_includes/nav_blog.html @@ -1,11 +1,15 @@ <div class="nav-docs nav-blog"> <div class="nav-docs-section"> - <h3>Recent posts</h3> <ul> - {% for post in site.posts limit:10 %} - <li><a href="{{ post.url }}"{% if page.title == post.title %} class="active"{% endif %}>{{ post.title }}</a></li> + {% for post in site.posts %} + <li> + <span class="post-date">{{ post.date | date: "%b %-d, %Y" }}</span> + <a class="post-link" href="{{ post.url | prepend: torch.ch/ }}">{{ post.title }}</a> + <br> + {{ post.excerpt }} + <img src="{{post.picture}}"></img> + </li> {% endfor %} - <li><a href="/blog/all.html">All posts ...</a></li> </ul> </div> </div> diff --git a/_layouts/post.html b/_layouts/post.html index 6e07161..7c980c4 100644 --- a/_layouts/post.html +++ b/_layouts/post.html @@ -7,9 +7,7 @@ id: blog <section class='content'><div class='width'> -{% include nav_blog.html %} - -<article class='withtoc'> +<article class='blogpost'> <h1>{{ page.title }}</h1> <!-- Look the author details up from the site config. --> {% assign author = site.data.authors[page.author] %} diff --git a/blog/_posts/2015-07-11-first-post.md b/blog/_posts/2015-07-11-first-post.md deleted file mode 100644 index 0b23114..0000000 --- a/blog/_posts/2015-07-11-first-post.md +++ /dev/null @@ -1,8 +0,0 @@ ---- -layout: post -title: First Torch blog post -comments: True -author: szagoruyko ---- - -This is a Torch blog. It is meant to post the latest news, additions and events in the Torch world. diff --git a/blog/_posts/2015-07-30-cifar.md b/blog/_posts/2015-07-30-cifar.md index 75bfbf5..60ab27d 100644 --- a/blog/_posts/2015-07-30-cifar.md +++ b/blog/_posts/2015-07-30-cifar.md @@ -3,6 +3,8 @@ layout: post title: 92.45% on CIFAR-10 in Torch comments: True author: szagoruyko +excerpt: CIFAR-10 is a popular vision dataset for trying out new ideas. We show that using a simple combination of Batch Normalization and a VGG-like convnet architecture, one can get a competitive baseline on the dataset. +picture: https://kaggle2.blob.core.windows.net/competitions/kaggle/3649/media/cifar-10.png --- <!---# 92.45% on CIFAR-10 in Torch--> diff --git a/blog/_posts/2015-09-07-spatial_transformers.md b/blog/_posts/2015-09-07-spatial_transformers.md new file mode 100644 index 0000000..e31f7f2 --- /dev/null +++ b/blog/_posts/2015-09-07-spatial_transformers.md @@ -0,0 +1,120 @@ +--- +layout: post +title: The power of Spatial Transformer Networks +comments: True +author: alband +excerpt: Spatial Transformers are an exciting new learnable layer that can be plugged into ConvNets. We show that using these layers in a ConvNet gets us to state of the art accuracy with a significantly smaller network. We also explore and visualize the learning that happens in the transform layers. +picture: https://raw.githubusercontent.com/moodstocks/gtsrb.torch/master/resources/st-mnist.png +--- + +<!---# The power of Spatial Transformer Networks; 99.61% on Traffic Signs with a small network.--> + +## tl;dr + +A few weeks ago, [Google DeepMind](http://deepmind.com/index.html) released an awesome paper called [Spatial Transformer Networks](http://arxiv.org/abs/1506.02025) aiming at boosting the geometric invariance of CNNs in a very elegant way. + +This approach was so appealing to us at [Moodstocks](https://moodstocks.com) that we decided to implement it and see how it performs on a not-so-simple dataset called the GTSRB. + +At the end of the day Spatial Transformer Networks enabled us to outperform the state-of-the-art with a much simpler pipeline (no jittering, no parallel networks, no fancy normalization techniques, ...) + +## The GTSRB dataset + +The GTSRB dataset (German Traffic Sign Recognition Benchmark) is provided by the Institut für Neuroinformatik group [here](http://benchmark.ini.rub.de/?section=gtsrb&subsection=news). It was published for a competition held in 2011 ([results](http://benchmark.ini.rub.de/?section=gtsrb&subsection=results)). Images are spread across 43 different types of traffic signs and contain a total of 39,209 train examples and 12,630 test ones. + + + +We like this dataset a lot at Moodstocks: it's lightweight, yet hard enough to test new ideas. For the record, the contest winner achieved a 99,46% top-1 accuracy thanks to **a committee of 25 networks** and by using a bunch of augmentations and data normalization techniques. + +## Spatial Transformer networks +The goal of spatial transformers [1] is to add to your base network a layer able to perform an explicit geometric transformation on an input. The parameters of the transformation are learnt thanks to the standard backpropagation algorithm, meaning there is no need for extra data or supervision. + +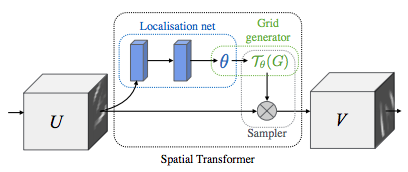 + +The layer is composed of 3 elements: + +* The *localization network* takes the original image as an input and outputs the parameters of the transformation we want to apply. +* The *grid generator* generates a grid of coordinates in the input image corresponding to each pixel from the output image. +* The *sampler* generates the output image using the grid given by the grid generator. + +As an example, here is what you get after training a network whose first layer is a ST: + +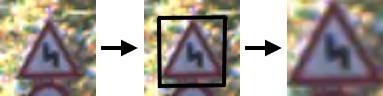 + +On the left you see the input image. In the middle you see which part of the input image is sampled. On the right you see the Spatial Transformer output image. + +## Results + +The [IDSIA](http://www.idsia.ch/) guys won the contest back in 2011 with a 99.46% top-1 accuracy. We achieved a *99.61% top-1 accuracy* with a much simpler pipeline: +<center> + +| Pipeline | IDSIA | Moodstocks | +| ------------- | --------- | ---------- | +| Augmentations | Yes (i) | No | +| Jittering | Yes (ii) | No | +| Network | ~90M weights (iii)| ~20M weights (iv)| +</center> + +* (i) 5 versions of the original dataset thanks to fancy normalization techniques +* (ii) scaling translations and rotations +* (iii) 25 networks with 3 convolutional layers and 2 fully connected layers each +* (iv) A single network with 3 convolutional layers and 2 fully connected layers + 2 spatial transformer layers + +## Interpretation + +Given these good results, we wanted to have some insights on which kind of transformations the Spatial Transformer is learning. Since we have a Spatial Transformer at the beginning of the network we can easily visualize its impact by looking at the transformed input image. + +### At training time + +Here the goal is to visualize how the Spatial Transformer behaves during training. + +In the animation below, you can see: + +* on the left the original image used as input, +* on the right the transformed image produced by the Spatial Transformer, +* on the bottom a counter that represents training steps (0 = before training, 10/10 = end of epoch 1). + +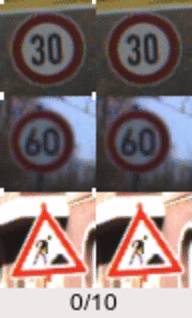 + +*Note: the white dots on the input image show the corners of the part of the image that is sampled. Same applies below.* + +As expected, we see that during the training, the Spatial Transformer learns to focus on the traffic sign, learning gradually to remove background. + +### Post-training + +Here the goal is to visualize the ability of the Spatial Transformer (once trained) to produce a stable output even though the input contains geometric noise. + +For the record the GTSRB dataset has been initially generated by extracting images from video sequences took while approaching a traffic sign. + +The animation below shows for each image of such a sequence (on the left) the corresponding output of the Spatial Transformer (on the right). + +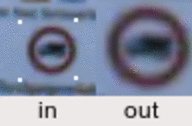 + +We can see that even though there is an important variability in the input images (scale and position in the image), the output of the Spatial Transformer remains almost static. + +This confirms the intuition we had on how the Spatial Transformer simplifies the task for the rest of the network: learning to only forward the interesting part of the input and removing geometric noise. + +The Spatial Transformer learned these transformations in an end-to-end fashion, without any modification to the backpropagation algorithm and without any extra annotations. + +## Code + +We leveraged the grid generator and the sampler coded by Maxime Oquab in his great [stnbhwd](https://github.com/qassemoquab/stnbhwd) project. We added a module placed between the localization network and the grid generator to let us restrict the possible transformations. + +Using these modules, creating a spatial transformer layer using torch logic is as easy as: + +{% gist albanD/954021a4be9e1ccab753 %} + +The full code is available on the [Moodstocks Github](https://github.com/moodstocks/gtsrb.torch). We designed it to let you perform a large range of tests on the dataset. If you are looking at reproducing our results, all you need is run the following command: + +``` bash +# This takes ~5 min per epoch and 1750MB ram on a Titan X +luajit main.lua -n -1 --st --locnet 200,300,200 --locnet3 150,150,150 --net idsia_net.lua --cnn 150,200,300,350 -e 14 +``` + +It will basically add two Spatial Transfomer layers (`--st --locnet 200,300,200 --locnet3 150,150,150`) to the baseline IDSIA network (`idsia_net.lua --cnn 150,200,300,350`) and run for 14 epochs (`-e 14`). Of course you can do much more with our code, so feel free to check out the [docs](https://github.com/moodstocks/gtsrb.torch#gtsrbtorch) in our repo! + +## Conclusion +Spatial Transformer Networks are a very appealing way to boost the geometric invariance of CNNs and hence improve your top-1 accuracy. They learn to account for geometric transformations relevant to your dataset without the need for extra supervision. Using them we managed to outperform the state-of-the-art on a not-so-simple dataset (GTSRB) while drastically simplifying the pipeline. Feel free to use [our code](https://github.com/moodstocks/gtsrb.torch) to reproduce our results or even get better ones: we provide a fancy way to [mass benchmark](https://github.com/Moodstocks/gtsrb.torch/blob/master/docs/bench.md) configurations to help you do that. Have fun! + +1. *Max Jaderberg, Karen Simonyan, Andrew Zisserman, Koray Kavukcuoglu*, Spatial Transformer Networks [[arxiv]](http://arxiv.org/abs/1506.02025) +2. *P. Sermanet, Y. LeCun*, Traffic sign recognition with multi-scale Convolutional Networks [[link]](http://yann.lecun.com/exdb/publis/pdf/sermanet-ijcnn-11.pdf) +3. *D. Ciresan, U. Meier, J. Masci, J. Schmidhuber*, Multi-column deep neural network for traffic sign classification [[link]](http://people.idsia.ch/~juergen/nn2012traffic.pdf) diff --git a/blog/all.md b/blog/all.md index 58d5e2a..d2e1c6b 100644 --- a/blog/all.md +++ b/blog/all.md @@ -7,5 +7,10 @@ id: blog ## Blog Posts {% for post in site.posts %} - * {{ post.date | date_to_string }} » [ {{ post.title }} ]({{ post.url }}) + <li> + <span class="post-date">{{ post.date | date: "%b %-d, %Y" }}</span> + <a class="post-link" href="{{ post.url | prepend: site.baseurl }}">{{ post.title }}</a> + <br> + {{ post.excerpt }} + </li> {% endfor %} diff --git a/blog/index.md b/blog/index.md index c159802..eccebbe 100644 --- a/blog/index.md +++ b/blog/index.md @@ -7,10 +7,4 @@ id: blog {% include nav_blog.html %} -{% for post in site.posts %} -### [ {{ post.title }} ]({{ post.url }}) -{% include post_detail.html %} - -{% endfor %} - {% include footer.html %} diff --git a/docs/02-developer-docs.md b/docs/02-developer-docs.md index 031ba81..60f73ee 100644 --- a/docs/02-developer-docs.md +++ b/docs/02-developer-docs.md @@ -7,9 +7,6 @@ previous: package-docs.html next: cvpr15.html --- -Developer Documentation -======================= - Writing your own nn modules =========================== @@ -23,60 +20,74 @@ If the module is heavier in computation, or you want to create specialized and optimized code for CPU or GPU, you might want to create the modules at the C / CUDA level (Section 2). -Modules are bricks to build neural networks. A Module is a neural network by itself, -but it can be combined with other networks using container classes to create -complex neural networks. Module is an abstract class which defines fundamental -methods necessary for a training a neural network. -All modules are serializable. +> Modules are bricks to build neural networks. A Module is a neural network by itself, but it can be combined with other networks using container classes to create complex neural networks. Module is an abstract class which defines fundamental methods necessary for a training a neural network. All modules are serializable. -Modules contain two states variables: output and gradInput. -Here we review the set of basic functions that a Module has to implement: +Modules contain two states variables: `output` and `gradInput`. +Here we review the set of basic functions that a `Module` has to implement: +```lua [output] forward(input) +``` + Takes an input object, and computes the corresponding output of the module. In general input and output are Tensors. However, some special sub-classes like table layers might expect something else. Please, refer to each module specification for further information. -After a forward(), the output state variable should have been updated to the new value. +After a `forward()`, the `output` state variable should have been updated to the new value. It is not advised to override this function. Instead, one should -implement updateOutput(input) function. -The forward(input) function in the abstract parent class Module will call updateOutput(input). +implement `updateOutput(input)` function. +The `forward(input)` function in the abstract parent class `Module` will call `updateOutput(input)`. +```lua [gradInput] backward(input, gradOutput) +``` + Performs a back-propagation step through the module, with respect to the given input. -In general this method makes the assumption forward(input) has been called before, with the same input. +In general this method makes the assumption `forward(input)` has been called before, with the same input. This is necessary for optimization reasons. -If you do not respect this rule, backward() will compute incorrect gradients. -In general input and gradOutput and gradInput are Tensors. -However, some special sub-classes like table layers might expect something else. +> If you do not respect this rule, `backward()` will compute incorrect gradients. + + +In general `input`, `gradOutput` and `gradInput` are `Tensors`. +However, some special sub-classes like `table` layers might expect something else. Please, refer to each module specification for further information. A backpropagation step consist in computing two kind of gradients at input -given gradOutput (gradients with respect to the output of the module). +given `gradOutput` (gradients with respect to the output of the module). This function simply performs this task using two function calls: -A function call to updateGradInput(input, gradOutput). -A function call to accGradParameters(input,gradOutput). +- A function call to `updateGradInput(input, gradOutput)`. +- A function call to `accGradParameters(input,gradOutput)`. + It is not advised to override this function call in custom classes. -It is better to override updateGradInput(input, gradOutput) and accGradParameters(input, gradOutput) functions. +It is better to override `updateGradInput(input, gradOutput)` and `accGradParameters(input, gradOutput)` functions. +```lua [output] updateOutput(input, gradOutput) +``` + When defining a new module, this method should be overloaded. Computes the output using the current parameter set of the class and input. -This function returns the result which is stored in the output field. +This function returns the result which is stored in the `output` field. +```lua [gradInput] updateGradInput(input, gradOutput) +``` + When defining a new module, this method should be overloaded. -Computing the gradient of the module with respect to its own input. -This is returned in gradInput. Also, the gradInput state variable is updated accordingly. +Computing the gradient of the module with respect to its own `input`. +This is returned in `gradInput`. Also, the `gradInput` state variable is updated accordingly. + +```lua +accGradParameters(input, gradOutput) +``` -[gradInput] accGradParameters(input, gradOutput) When defining a new module, this method may need to be overloaded, if the module has trainable parameters. Computing the gradient of the module with respect to its own parameters. @@ -84,15 +95,18 @@ Many modules do not perform this step as they do not have any parameters. The state variable name for the parameters is module dependent. The module is expected to accumulate the gradients with respect to the parameters in some variable. -Zeroing this accumulation is achieved with zeroGradParameters() and -updating the parameters according to this accumulation is done with updateParameters(). +Zeroing this accumulation is achieved with `zeroGradParameters()` and +updating the parameters according to this accumulation is done with `updateParameters()`. +```lua reset() +``` + This method defines how the trainable parameters are reset, i.e. initialized before training. Modules provide a few other methods that you might want to define, -if you are not planing to use the optim package. -These methods help zero() the parameters, and update them using very basic techniques. +if you are not planning to use the `optim` package. +These methods help `zero()` the parameters, and update them using very basic techniques. In terms of code structure, Torch provides a class model, which we use for inheritance, and in general for the definition of all the modules in nn. @@ -120,7 +134,7 @@ end ``` When defining a new class, all we need to do is fill in these empty functions. -Note that when defining the constructor __init(), we always call the parent's constructor first. +Note that when defining the constructor `__init()`, we always call the parent's constructor first. Let's see some practical examples now. @@ -128,12 +142,198 @@ Let's see some practical examples now. 1. Writing modules at the Lua level: Implementing Dropout Activation Units ======================================================================= +Dropout units have a central idea there is to perturbate the activations of hidden units, by randomly zeroing some of these units. +Such a class could be defined like this: +```lua +local Dropout, Parent = torch.class('nn.Dropout', 'nn.Module') +function Dropout:__init(p) + Parent.__init(self) + self.p = p or 0.5 + if self.p >= 1 or self.p < 0 then + error('<Dropout> illegal percentage, must be 0 <= p < 1') + end + self.noise = torch.Tensor() +end +function Dropout:updateOutput(input) + self.output:resizeAs(input):copy(input) + self.noise:resizeAs(input) + self.noise:bernoulli(1-self.p) + self.output:cmul(self.noise) + return self.output +end +function Dropout:updateGradInput(input, gradOutput) + self.gradInput:resizeAs(gradOutput):copy(gradOutput) + self.gradInput:cmul(self.noise) -- simply mask the gradients with the noise vector + return self.gradInput +end +``` + +When writing modules with gradient estimation, it's always very important to test your implementation. This can be easily done using the `Jacobian` class provided in `nn`, which compares the implementation of the gradient methods (`updateGradInput()` and `accGradParameters()`) with the `Jacobian` matrix obtained by finite differences (perturbating the input of the module, and estimating the deltas on the output). This can be done like this: +```lua +-- parameters +local precision = 1e-5 +local jac = nn.Jacobian + +-- define inputs and module +local ini = math.random(10,20) +local inj = math.random(10,20) +local ink = math.random(10,20) +local percentage = 0.5 +local input = torch.Tensor(ini,inj,ink):zero() +local module = nn.Dropout(percentage) + +-- test backprop, with Jacobian +local err = jac.testJacobian(module,input) +print('==> error: ' .. err) +if err<precision then + print('==> module OK') +else + print('==> error too large, incorrect implementation') +end +``` + +One slight issue with the `Jacobian` class is the fact that it assumes that the outputs of a module are deterministic wrt to the inputs. This is not the case for that particular module, so for the purpose of these tests we need to freeze the noise generation, i.e. do it only once: + +-- we overload the updateOutput() function to generate noise only +-- once for the whole test. + +```lua +function Dropout:updateOutput(input) + self.output:resizeAs(input):copy(input) + self.noise = self.noise or input.new():resizeAs(input):bernoulli(1-self.p) + self.output:cmul(self.noise) + return self.output +end +``` 2. Writing modules at the C or CUDA level ========================================= + +## C macro based templates + +Before writing Torch C code, one has to first get familiar with the C macro syntax that is sprinkled all over Torch and nn. + +For example, look at this code that appears in [THTensorMath.c](https://github.com/torch/torch7/blob/c55a0621ae5f306fcd4edf03bd382dd3729972d9/lib/TH/generic/THTensorMath.c#L374-L388) + +```C +void THTensor_(add)(THTensor *r_, THTensor *t, real value) +{ + THTensor_(resizeAs)(r_, t); + if (THTensor_(isContiguous)(r_) && THTensor_(isContiguous)(t) && THTensor_(nElement)(r_) == THTensor_(nElement)(t)) { + real *tp = THTensor_(data)(t); + real *rp = THTensor_(data)(r_); + long sz = THTensor_(nElement)(t); + long i; + #pragma omp parallel for if(sz > TH_OMP_OVERHEAD_THRESHOLD) private(i) + for (i=0; i<sz; i++) + rp[i] = tp[i] + value; + } else { + TH_TENSOR_APPLY2(real, r_, real, t, *r__data = *t_data + value;); + } +} +``` + +The strange `_(add)(THTensor *r_ ....)` syntax that you see is a preprocessor macro. + +```C +lib/TH/THTensor.h: +#define THTensor_(NAME) TH_CONCAT_4(TH,Real,Tensor_,NAME) +``` +which leads to... + +```C +lib/TH/THGeneral.h.in: +#define TH_CONCAT_4(x,y,z,w) TH_CONCAT_4_EXPAND(x,y,z,w) +``` +and finally... + +```C +lib/TH/THGeneral.h.in: +#define TH_CONCAT_4_EXPAND(x,y,z,w) x ## y ## z ## w +``` + +Therefore, (and after preprocessing with a few more macros), + +```C +void THTensor_(add)(THTensor *r_, THTensor *t, real value) +``` + +ultimately becomes this: + +```C +long THRealTensor_add(const THRealTensor *r_, THRealTensor *t, real value) +``` + +Real and real are defined to be of a specific type, for example, for float precision: + +```C +#define Real Float +#define real float +``` + +finally makes that function prototype: + +```C +long THFloatTensor_add(const THFloatTensor *r_, THFloatTensor *t, float value) +``` + +[Aren't preprocessors just grand ?](http://stackoverflow.com/questions/30420807/strange-c-syntax-in-lua-library) + +You will see similar syntax in the nn library as well, so brace yourself well for this syntax. + + +## C nn module + +The best way to understand how to write a new nn module is by looking at an existing one. + +Here is a walkthrough of writing nn.Threshold: + +### Step 1: write the Lua part + +https://github.com/torch/nn/blob/master/Threshold.lua + +- write the constructor +- write updateOutput / updateGradInput that simply call into C + +Calling into C has an efficient but slightly weird syntax: + +https://github.com/torch/nn/blob/b80e26e8b849a69b8121acf62f3487095c2f10e8/Threshold.lua#L20 + +```lua +input.nn.Threshold_updateOutput(self, input) +``` + +This line is simply calling the function defined here: +https://github.com/torch/nn/blob/b80e26e8b849a69b8121acf62f3487095c2f10e8/generic/Threshold.c#L5 + +And the reason it calls that is because you register the C function under the input.nn. table here: https://github.com/torch/nn/blob/b80e26e8b849a69b8121acf62f3487095c2f10e8/generic/Threshold.c#L61-L63 + +This helps us write generic code that works for any defined tensor type, while not doing any complicated dynamic function dispatch logic. + +The complete nn.Threshold module is written as two files: +- Lua part: https://github.com/torch/nn/blob/b80e26e8b849a69b8121acf62f3487095c2f10e8/Threshold.lua +- C part: https://github.com/torch/nn/blob/b80e26e8b849a69b8121acf62f3487095c2f10e8/generic/Threshold.c + +The files are included into the package at these lines: +- init.lua : https://github.com/torch/nn/blob/b80e26e8b849a69b8121acf62f3487095c2f10e8/init.lua#L68 +- init.c : https://github.com/torch/nn/blob/b80e26e8b849a69b8121acf62f3487095c2f10e8/init.c#L41-L42 +- init.c : https://github.com/torch/nn/blob/b80e26e8b849a69b8121acf62f3487095c2f10e8/init.c#L153 +- init.c : https://github.com/torch/nn/blob/b80e26e8b849a69b8121acf62f3487095c2f10e8/init.c#L194 + +## CUDA nn module + +To add CUDA support to the nn.Threshold module, similar to writing a Threshold.c, we will write a Threshold.cu + +- https://github.com/torch/cunn/blob/master/Threshold.cu + +and include it here: + +- https://github.com/torch/cunn/blob/master/init.cu#L36 +- https://github.com/torch/cunn/blob/master/utils.h#L30 + diff --git a/docs/whoweare.md b/docs/whoweare.md index 61e2702..ef42ba5 100644 --- a/docs/whoweare.md +++ b/docs/whoweare.md @@ -14,7 +14,7 @@ permalink: /whoweare.html ## Major Community Contributors [Nicholas Leonard - Research Engineer @ Element Inc](https://github.com/nicholas-leonard) -[Jonathan Tompson - PhD candidate @ NYU](http://www.cims.nyu.edu/~tompson/) +[Jonathan Tompson - Research Scientist @ Google](http://www.cims.nyu.edu/~tompson/) [Sergey Zagoruyko - PhD candidate @ IMAGINE](http://imagine.enpc.fr/~zagoruys/) [Aysegul Dundar, Jonghoon Jin, Alfredo Canziani - e-Lab Purdue and TeraDeep Inc.](https://engineering.purdue.edu/elab/) diff --git a/static/flow.css b/static/flow.css index c96a98a..ad99d39 100644 --- a/static/flow.css +++ b/static/flow.css @@ -14,13 +14,12 @@ html { } body { - font-family: "Helvetica Neue", Helvetica, Arial, sans-serif; - font-family: proxima-nova, "Helvetica Neue", Helvetica, Arial, sans-serif; - font-weight: 300; + font-family: "Helvetica Neue", Helvetica, "Segoe UI", Arial, freesans, sans-serif; + font-size: 16px; color: #444; margin: 0; padding-top: 50px; - line-height: 22px; + line-height: 1.6; } @@ -45,7 +44,10 @@ h1 { } h2 { - font-size: 1.5em; + padding-bottom: 0.3em; + font-size: 1.75em; + line-height: 1.225; + border-bottom: 1px solid #eee; } h3 { @@ -348,6 +350,10 @@ section.content article.withtoc { position: relative; } +section.content article.blogpost { + +} + section.content aside .fb-like { top: 4px; left: 4px; @@ -501,7 +507,7 @@ div.CodeMirror-lines { section.content article { margin: 0 auto; - width: 860px; + width: 800px; } section.content nav.toc { |